Developing a dishwasher liquid requires more than checking to see how clean the dishes are after it’s used. A leading global Consumer Packaged Goods (CPG) company uses analytics to predict how moisture would interact with certain scents, so that throughout the dishwashing process, consumers get the right smell at the optimal time.
Fast-food chains need to keep an eye on more than just successful promotions and in-demand products. A leading fast-food chain monitors the design of its drive-through, information provided to customers and their resulting orders and engagement. Analytics helps spot issues with the service, and ultimately ensures the company serves each of its 69-million daily customers consistently.
These companies are successfully leveraging analytics to drive impactful outcomes. Here, we look at some of the emerging trends in this space that can help businesses stay ahead of the game like these companies.
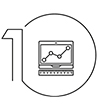
Augmented Anlaytics
Augmented analytics is an approach that relies on Machine Learning (ML) and Natural Language Processing (NLP)1 to help businesses obtain actionable insights more easily. The term was coined by Gartner in 2017, and since then businesses have been exploring the potential of augmented analytics to enhance existing data analytics processes, and changing the way businesses develop, consume and share analytics content.
While it is well-understood that analytics can help businesses, the process for it to work can be challenging as businesses have to collect data from a variety of sources, clean this data, conduct the analysis and get to a point where they have insights which they can act on.
By integrating augmented analytics to existing data analytics software, businesses can simplify the process. Augmented analytics can handle large data sets, and even scrub and parse raw data without the need for pricey and hard-to-find data scientists to work on these time-intensive tasks. This also means that the data scientists can spend the majority of their time on delivering value to the business by searching for actionable insights.
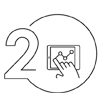
Explainable AI
We have all heard of the many benefits of Artificial Intelligence (AI) and PwC2 suggests that the technology is a transformational USD 15-Trillion opportunity. But as more and more decision-making will be made by an algorithmic ‘black box,’ there has to be a high level of confidence in the process itself.
‘Explainable AI’ is essentially understanding the rationale of how the algorithm arrived at its recommendation or decision in the first place. This is not as easy as it sounds, as most AI models cannot explain how they got to a specific decision. Gartner explains that ‘Explainable AI’ in data science and ML platforms auto-generates an explanation of models, with information on accuracy, attributes, model statistics and features in natural language.
Explainable AI could be particularly useful if a business suffers negatively after making a decision based on AI. The business can then check whether the AI was working correctly and, if required, alter the algorithm, or get insights on whether an external factor led to the negative impact instead. Explainable AI could also prove useful to keep an eye on the ethical side of doing business. For example, with candidate screening, if an AI solution continuously hires people of a certain race and gender, there could be some bias in the algorithm. With Explainable AI, the business can check whether this is the case or whether it is a coincidence.
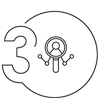
Subjective Analytics
A big focus for companies has been on understanding and optimizing the customer experience. Businesses currently analyze structured data from operational systems and unstructured data such as texts, images and sensors into workflows. However, the next step could be to take into account both operational data and the subjective experiences of individuals.
For example, if a user is frustrated by a particular experience or product there would be a way of feeding this into an overarching analytics platform. If it is clear that a customer has tried to engage in various ways with a company on numerous occasions without being able to resolve an issue, this should be factored into the way the business interacts with them. Perhaps a customer does not like the options they have in dealing with an issue, even though 95 percent of customers in that segment are satisfied with it. This added layer of subjective analytics can help businesses personalize customer experiences further.
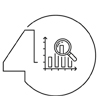
Graph Analytics
Graph analytics is used to analyze the relationships between different organizations, objects, people, transactions or even nodes in a network. By doing this, enterprises can check the extent of the correlation between different entities, and how often they engage with one another, and whether or not there are additional entities that alter this dynamic. Furthermore, businesses can verify which entities initiate the engagement, whether it is a one-way engagement or whether one entity always leads the conversation.
Gartner3 believes that graph analytics will become a necessity in the next few years for some enterprises when they ask difficult questions around complicated data.
One of the key areas for the use of graph analytics is within social media networks. Facebook’s graph search capability enables people to search for friends who prefer the same hockey team, while LinkedIn uses the same technology to help business professionals expand their networks. However, while this could be carried out using other technologies such as ML, graph analytics helps a business that is delving into this data to find out more about a specific relationship. It can help mine the relationship data to find out a person’s associations, likely political views and other interests. It can then target them with tailored advertising with higher chances of engagement.
Meanwhile, brands could also use graph analytics to find out which influencers yield the most social power, negate cyber threats by spotting anomalies in the way two entities engage with one another, and even accelerate post-merger sales.4
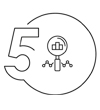
Compliance Analytics
Strategies that center around data have become imperative for businesses striving to digitally transform. However, while there are numerous benefits of data analytics, there are also precautions businesses need to take around security, privacy and ethics. Often, due to the added complexity of the technologies used, it is harder for businesses to know when they have breached regulatory norms. With compliance analytics, businesses can take preventive measures against high-profile events such as data breaches, questionable sales practices and organizational misconduct.
According to KPMG’s 2019 Chief Compliance Officers (CCOs) Survey,5 there are five key areas where CCOs are planning enhancements to their enterprise-wide ethics and compliance activities. Data analytics (32 percent) ranks third in that list.
Ernst & Young6 terms this as Forensic Data Analytics (FDA) that helps companies identify patterns of data, or information deduced from multiple data sources, that require closer attention for risk control purposes. FDA can help businesses scan an entire data set instead of relying on a sample data set that may not always give a clear picture.
For example, if a business believes an insider might have accessed or compromised sensitive data, it could apply FDA across multiple sets of data, including network access logs. If suspicious patterns are identified, senior executives can then put into place investigative procedures.
The above trends suggest that analytical tools are becoming more sophisticated and specialized. Business leaders will have to work with data experts to better understand the benefits of deploying the right analytical tools for their business to be a step ahead of the competition.
Click here to view the infographic
References:
-
https://www.gartner.com/smarterwithgartner/top-trends-in-the-gartner-hype-cycle-for-emerging-technologies-2017/
-
https://www.pwc.co.uk/services/audit-assurance/risk-assurance/services/technology-risk/technology-risk-insights/explainable-ai.html
-
https://www.gartner.com/en/newsroom/press-releases/2019-02-18-gartner-identifies-top-10-data-and-analytics-technolo
-
https://www.cmswire.com/analytics/how-social-graph-analytics-help-accelerate-post-merger-sales/
-
https://advisory.kpmg.us/articles/2019/2019-cco-survey-ungated.html
-
. https://www.ey.com/en_gl/trust/gdpr-compliance-how-data-analytics-can-help